TL;DR: Managing large-scale visual repositories is challenging due to data quality issues, silos, and high operational costs. Visual Layer’s VL Profiler, uses a robust graph-based engine, identifies and clusters data anomalies, reducing manual labor. It also supports the release of VL Datasets, created using VL Profiler to provide clean data for the community to train better AI /generative AI models. This system is designed to scale, handling up to billions of images, with more features to come.
The Messy World of Visual Data
In the rapidly evolving world of artificial intelligence, managing large-scale visual repositories has become a significant challenge. If you’ve ever worked with large-scale visual repositories, you’re likely familiar with the struggles of manual work and in-house tooling.
The sheer volume of visual data combined with inadequate tooling for the task make day-to-day tasks such as ensuring data quality, identifying anomalies and organizing data nearly impossible tasks. This has multiple implications:
1. Low model and product quality
The above not only hinders productivity but also produces suboptimal AI models and error-prone results that eventually lead to low product and service quality. For example, if you are training your model on the popular academic dataset LAION-1B, you are using nearly 105M low-quality images.
2. Data silos
According to Gartner, unstructured data now represents 80–90% of all new enterprise data, but just 18% of organizations are taking advantage of this data. Whether stored locally or in the cloud, data is dispersed across numerous locations. This lack of easy access and visibility obstructs efficient data usage and inhibits potential growth.
3. High operational costs
The manual process of labeling and reviewing images can cost up to several dollars per image, and this doesn’t even include the added expenses for storage, computing, and labor. According to Gartner, poor data quality costs organizations an average $12.9 million every year. This high operational cost can drain resources, stifling business growth and undermining profitability.
Introducing: VL Profiler
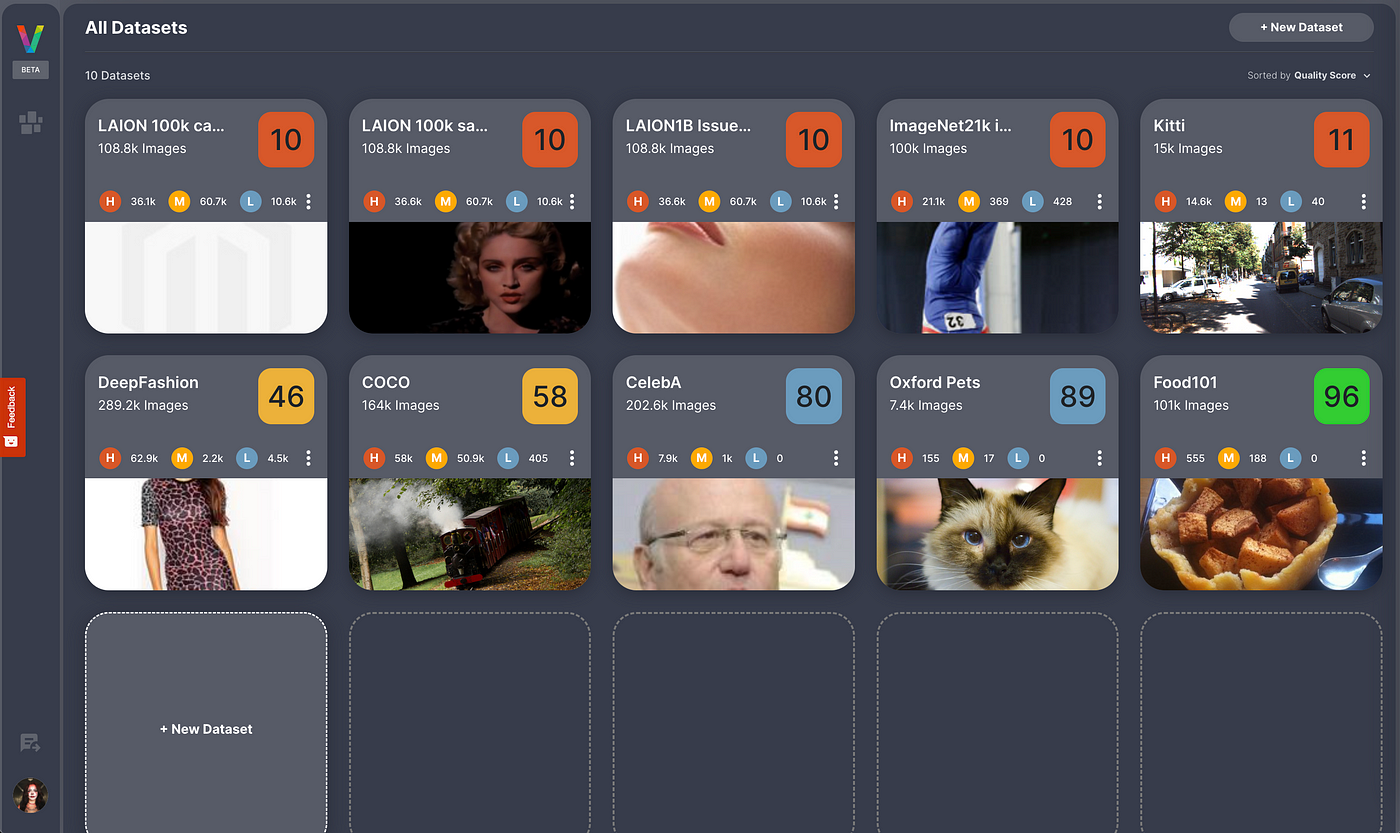
Visual Layer is building a single access layer for all visual data that allows you to seamlessly explore, search, and organize vast amounts of images and videos with ease. That’s why we’re excited to introduce VL Profiler, a groundbreaking solution designed to revolutionize the way you handle visual data and establish data quality standards for it.
VL Profiler is built upon the same robust and scalable graph-based engine behind fastdup, trusted by more than 220K users across the world and is used to process tens of thousands of datasets a day.
VL Profiler quickly and accurately identifies data anomalies and quality issues within your visual repositories. By clustering these issues into logical groups, Profiler enables you to address numerous images simultaneously, eliminating the need for tedious individual handling.
Key Features:
1. Fast and accurate data issues identification
A graph-based engine that leverages cutting-edge algorithms to swiftly and precisely identify anomalies and quality problems within your visual data, on both images and contained objects. From mislabeled images to outliers and duplicates, Profiler helps you remove redundant and low-quality data and correct annotations associated with it.
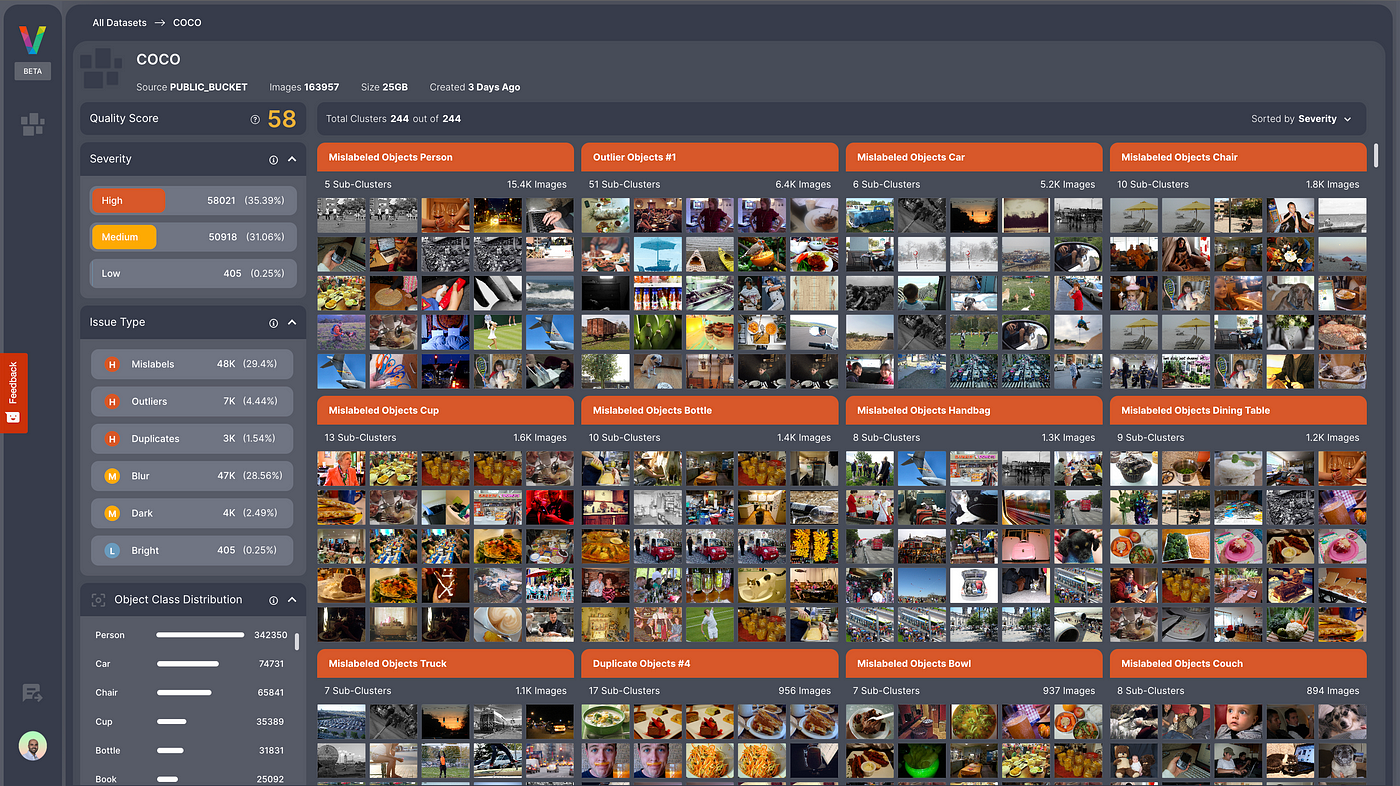
2. Logical grouping
You can say goodbye to manually sifting through individual images. By clustering related anomalies into logical groups, Profiler enables you to address issues at scale, significantly reducing manual effort and saving valuable time.
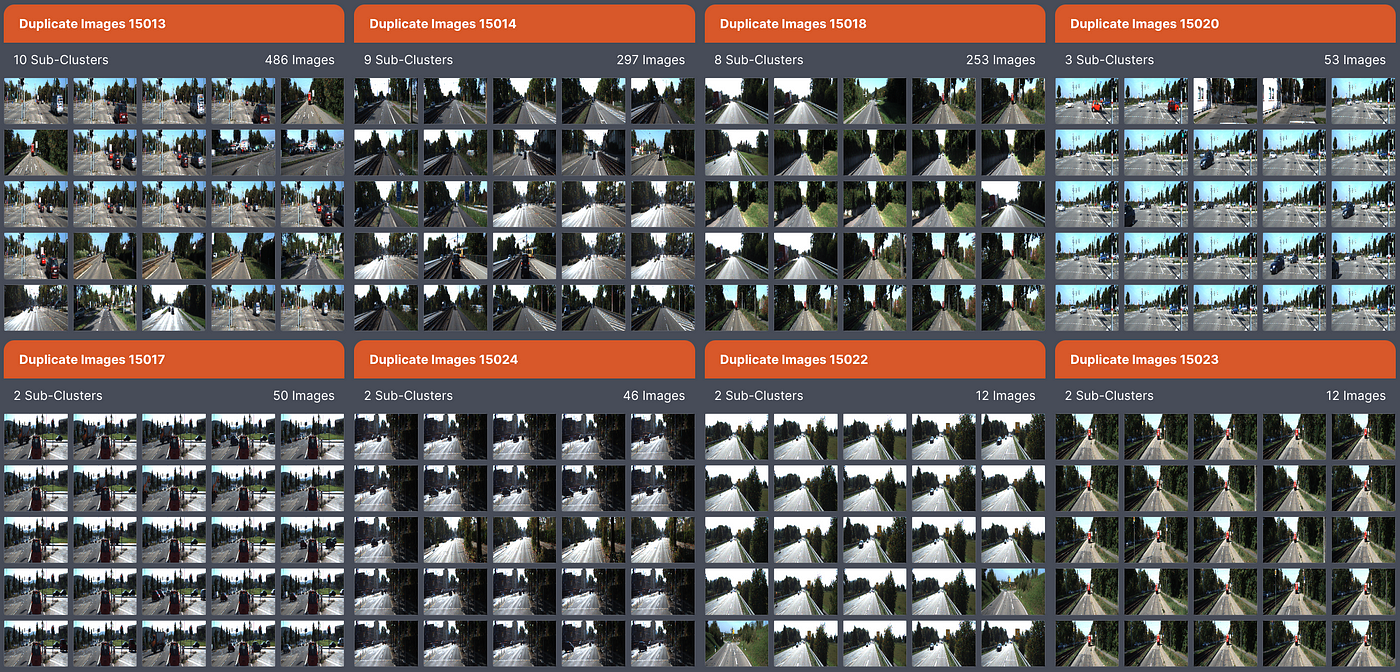

3. Unparalleled scale
Designed and built to scale from the ground up, Profiler ensures the flexibility necessary to meet your expanding data requirements. You can perform actions such as scanning, searching, and exploring large-scale data repositories, accommodating up to billions of images with ease and all of the image/object annotations associated with them.
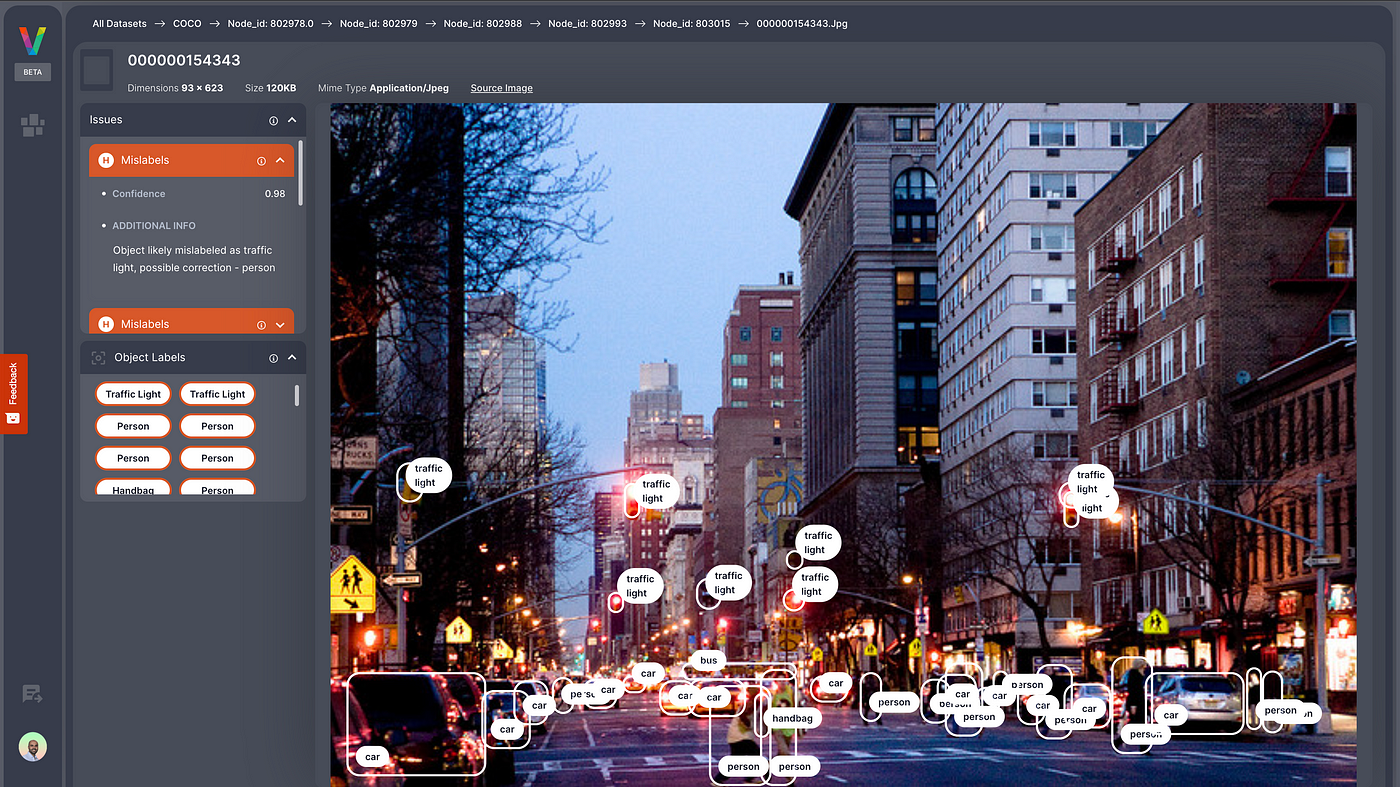
Coming Soon:
1. Actionable results
Viewing data quality issues is only the beginning, undertaking corrective actions on a multitude of images simultaneously, encompassing tasks such as eliminating redundant and low-quality images, as well as forwarding images for re-labeling is absolutely awesome.
2. Additional integrations
Currently, you can upload data locally to Profiler or from a public S3 bucket. Soon more data source integrations will follow, including an expansion to its native AWS integration which will also support private buckets. Another type of integration that is coming soon is with Image Annotations providers, such as Labelbox.
3. Image exploration and search
Profiler now offers a powerful view into the issues in your data, but soon it will also offer you unique exploration and search capabilities for all of your data, regardless of where it is stored.
Introducing VL Datasets:
Using VL Profiler we created VL Datasets to be free and open-source as a way of giving back to the community. VL Datasets can be used as a starting point to train your machine learning model on clean datasets. One of the publised free VL Datasets is Laion-1B (which contains 1 Billion images), where we have found nearly 105,000,000 duplicated images and many additional quality issues such as outliers and blurry images.
By utilizing VL Pofiler graph-based engine to identify and cluster data anomalies and quality issues we eliminate the need for tedious individual and manual task of isolating these issues. Read more about VL Dataset on this blog post.
Conclusion:
Say goodbye to the messy and labor-intensive management of large-scale visual repositories. With VL Profiler, you can revolutionize the way you manage and leverage your digital assets.
VL Profiler helps you Improve your data model, streamline your application development, slash labeling and cloud costs and maximize the utilization of your unstructured data.
Use Profiler from the web application or the python SDK.
To learn more about VL Profiler and how it can transform your visual data management, visit our website and get started for free today.
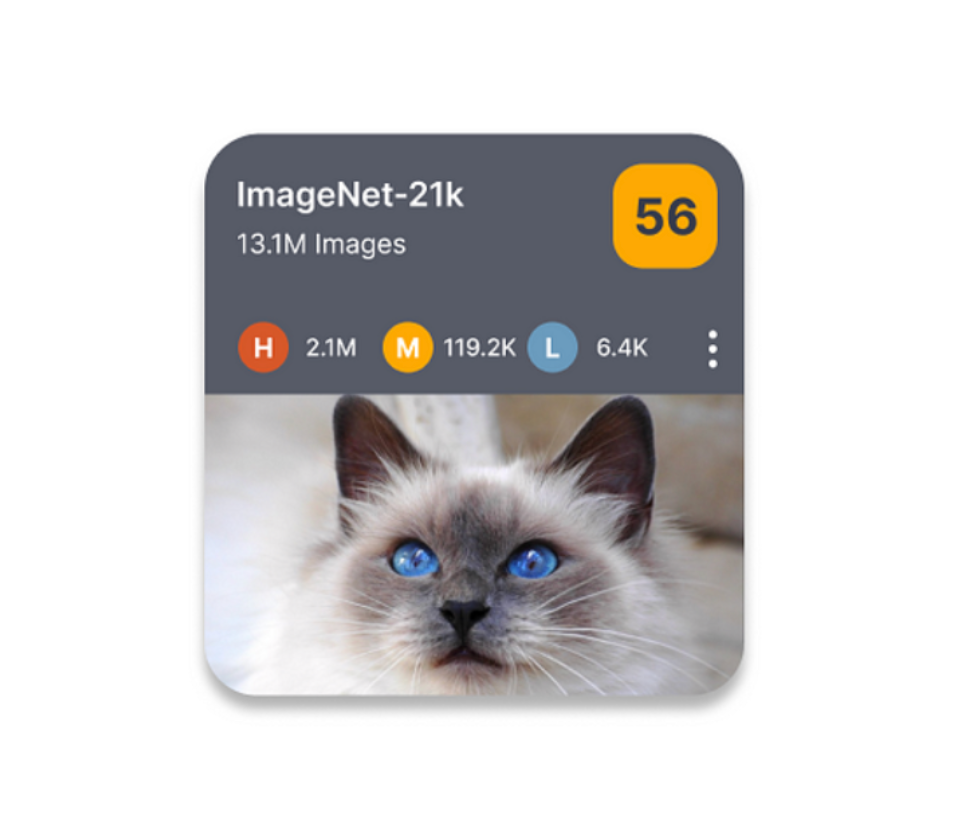
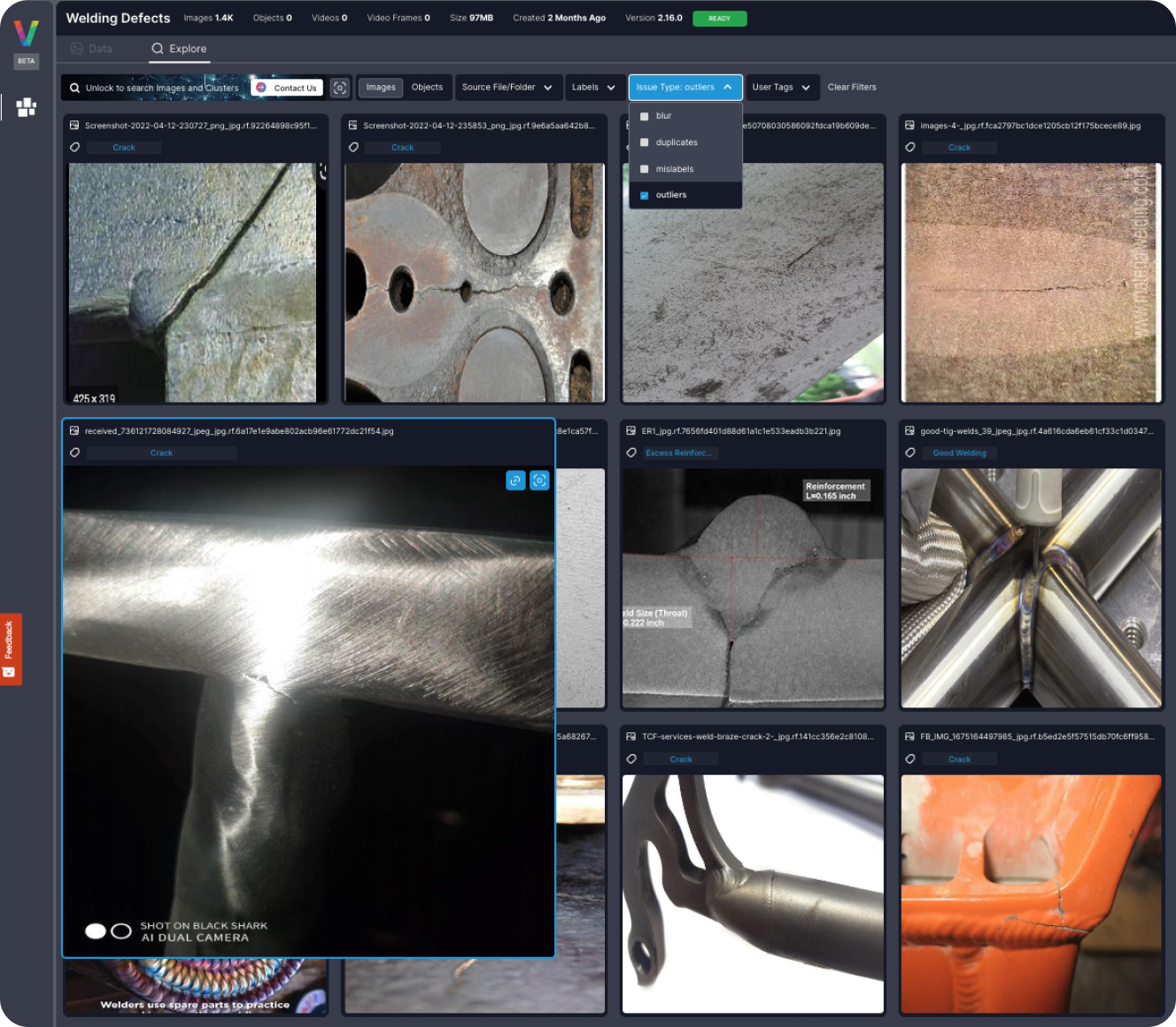
Introduction to Image Captioning
h2
h3
Image Captioning is the process of using a deep learning model to describe the content of an image. Most captioning architectures use an encoder-decoder framework, where a convolutional neural network (CNN) encodes the visual features of an image, and a recurrent neural network (RNN) decodes the features into a descriptive text sequence.
VQA
Visual Question Answering (VQA) is the process of asking a question about the contents of an image, and outputting an answer. VQA uses similar architectures to image captioning, except that a text input is also encoded into the same vector space as the image input.
code
Image captioning and VQA are used in a wide array of applications:
- point
- point
- point
Why Captioning With fastdup?
Image captioning can be a computationally-expensive task, requiring many processor hours to conduct. Recent experiments have shown that the free fastdup tool can be used to reduce dataset size without losing training accuracy. By generating captions and VQAs with fastdup, you can save expensive compute hours by filtering out duplicate data and unnecessary inputs.
quote
Getting Started With Captioning in fastdup
To start generating captions with fastdup, you’ll first need to install and import fastdup in your computing environment.
Processor Selection and Batching
The captioning method in fastdup enables you to select either a GPU or CPU for computation, and decide your preferred batch size. By default, CPU computation is selected, and batch sizes are set to 8. For GPUs with high-RAM (40GB), a batch size of 256 will enable captioning in under 0.05 seconds per image.
To select a model, processing device, and batch size, the following syntax is used. If no parameters are entered, the fd.caption() method will default to ViT-GPT2, CPU processing, and a batch size of 8.
“The captioning method in fastdup enables you to select either a GPU or CPU for computation, and decide your preferred batch size. By default, CPU computation is selected, and batch sizes are set to 8. For GPUs with high-RAM (40GB), a batch size of 256 will enable captioning in under 0.05 seconds per image.”
Dean Scontras, AVP, Public Sector, Wiz
FedRAMP is a government-wide program that provides a standardized approach to security in the cloud, helping government agencies accelerate cloud adoption with a common security framework. Achieving a FedRAMP Moderate authorization means Wiz has gone under rigorous internal and external security assessment to show it meets the security standards of the Federal Government and complies with required controls from the National Institute of Standards and Technology (NIST) Special Publication 800-53.
Image captioning and VQA are used in a wide array of applications:
- ⚡ Quickstart: Learn how to install fastdup, load a dataset, and analyze it for potential issues such as duplicates/near-duplicates, broken images, outliers, dark/bright/blurry images, and view visually similar image clusters. If you’re new, start here!
- 🧹 Clean Image Folder: Learn how to analyze and clean a folder of images from potential issues and export a list of problematic files for further action. If you have an unorganized folder of images, this is a good place to start.
- 🖼 Analyze Image Classification Dataset: Learn how to load a labeled image classification dataset and analyze for potential issues. If you have labeled ImageNet-style folder structure, have a go!
- 🎁 Analyze Object Detection Dataset: Learn how to load bounding box annotations for object detection and analyze for potential issues. If you have a COCO-style labeled object detection dataset, give this example a try.