Introducing VL Datasets
Open and Free Clean Image Datasets - A Game-Changer for Generative AI and Beyond.


TL;DR: Generative AI models heavily rely on clean, large-scale image datasets. However, issues such as duplicates and mislabels plague existing datasets like LAION-1B. To combat this, we introduce VL Datasets, a collection of clean datasets for Visual AI applications, created using Visual Layer’s newly introduced VL Profiler. We are releasing a cleaned-up version of some of the most popular visual datasets such as LAION-1B & ImageNet-21K. These datasets, we dub VL Datasets are accessible for free via the visuallayer Python SDK or via the VL Profiler UI, aiding in more robust and reliable AI model development.
Introduction
Generative AI, a technology that revolutionized the fields of art and design among others has gained significant popularity in recent years. This technology, however, heavily relies on high-quality, large-scale image datasets to train robust models. Whether you’re a researcher, student, data scientist, or an AI enthusiast, you’ve likely encountered challenges with messy image datasets in Generative AI or other AI applications involving visual data.
These challenges, such as duplicated images, mislabeled images, or outliers, can significantly impact the reliability of your models, waste storage and compute resources and take up valuable time and effort to clean up manually ¹.
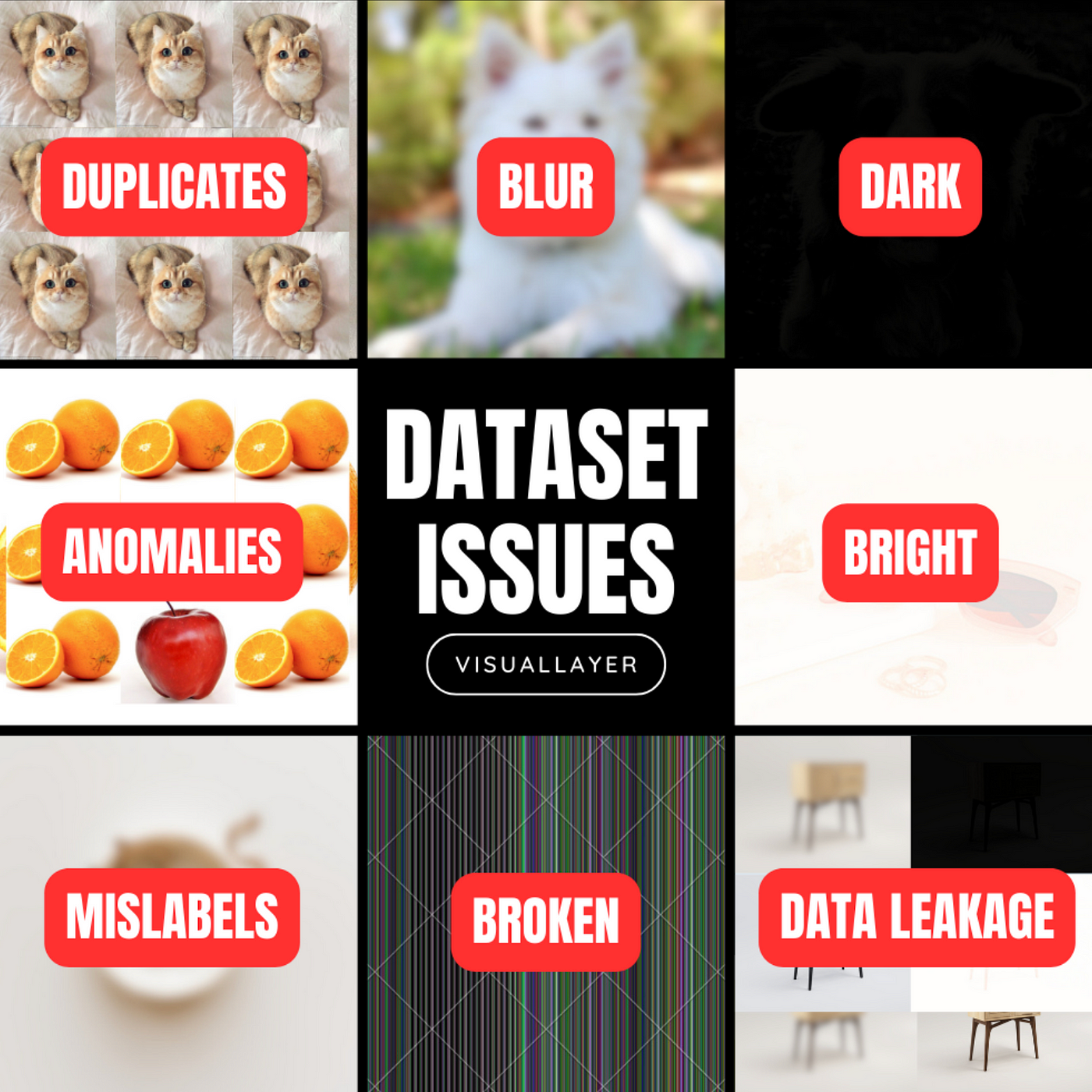
Revisiting The Problem
Handling large-scale image datasets is complicated. Common issues such as mislabels, duplicates, and outliers can compromise up to 46% of a dataset, leading to suboptimal models and wasted resources ¹.
Despite the critical role of these datasets in diverse applications, the tools for managing visual data often lack the necessary features, and teams are compelled to write their own custom tools ¹ .
We analyzed several open-source widely used academic datasets such as ImageNet-1K, ImageNet-21K, and LAION-1B and found that the datasets are plagued with various quality issues.
In LAION-1B we found that nearly 105M of the images have quality issues. For instance, more than 90M of the images have duplicate copies, more than 7M images are blurry or low quality and more than 6M images are considered outliers.
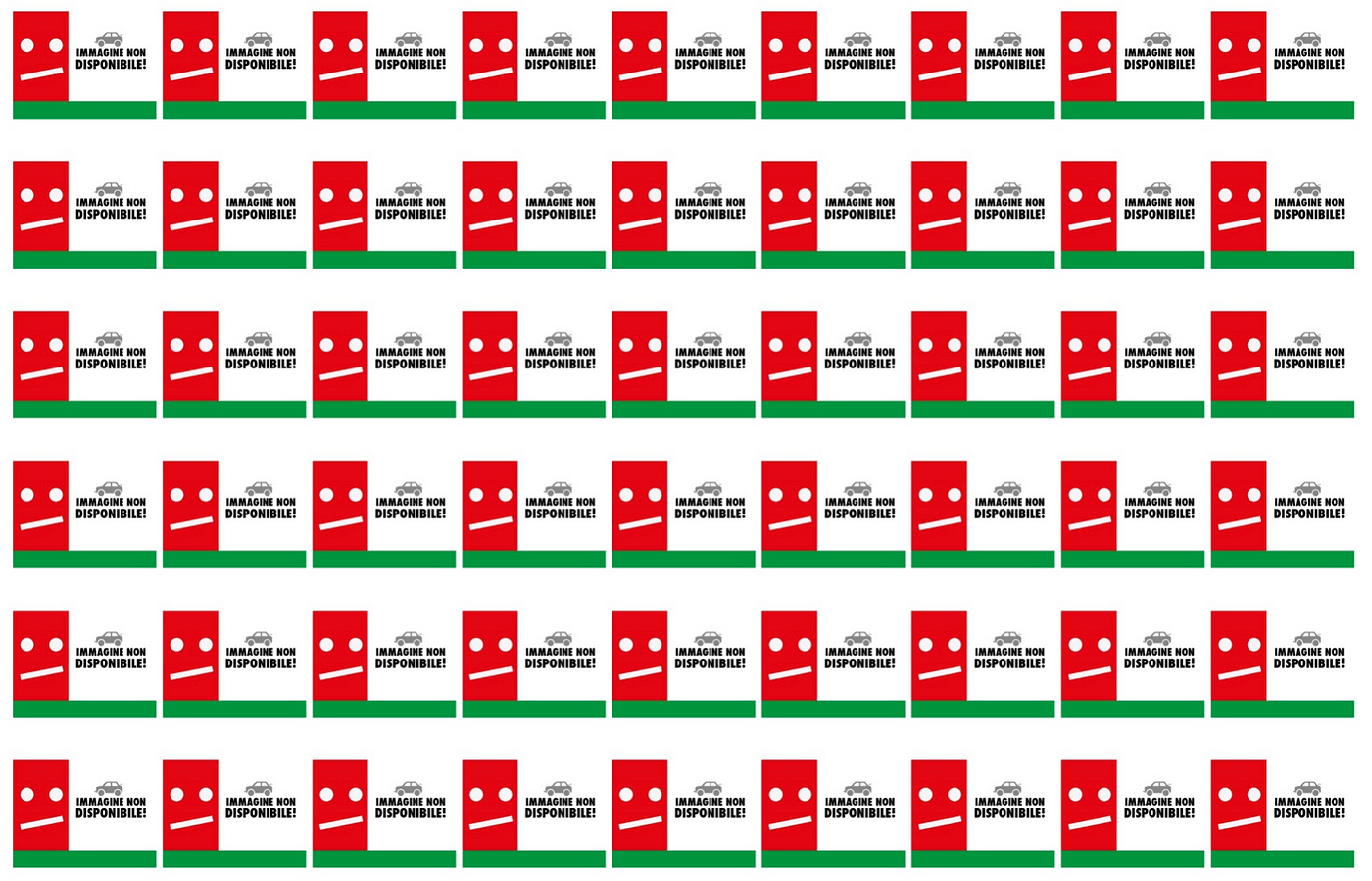
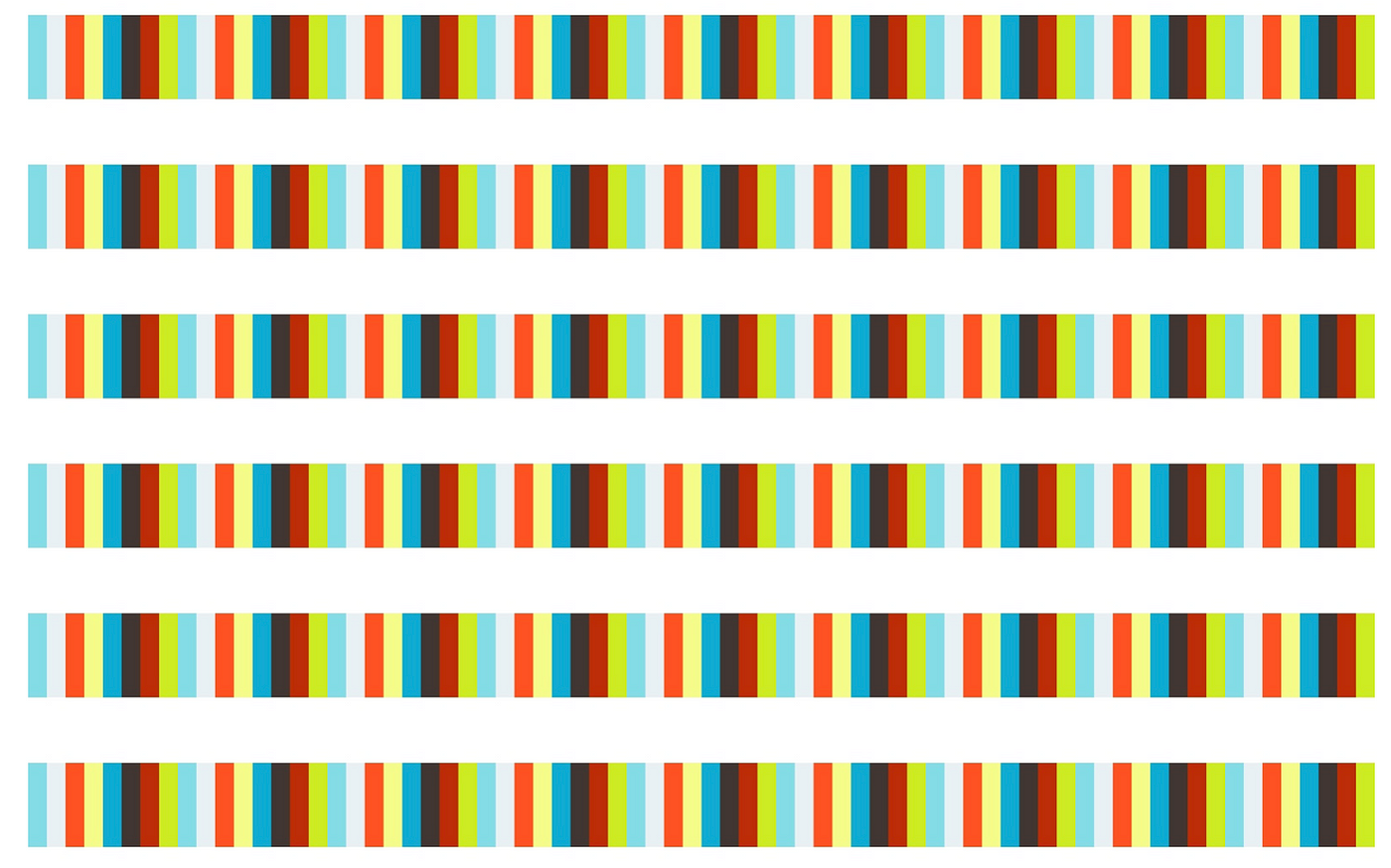
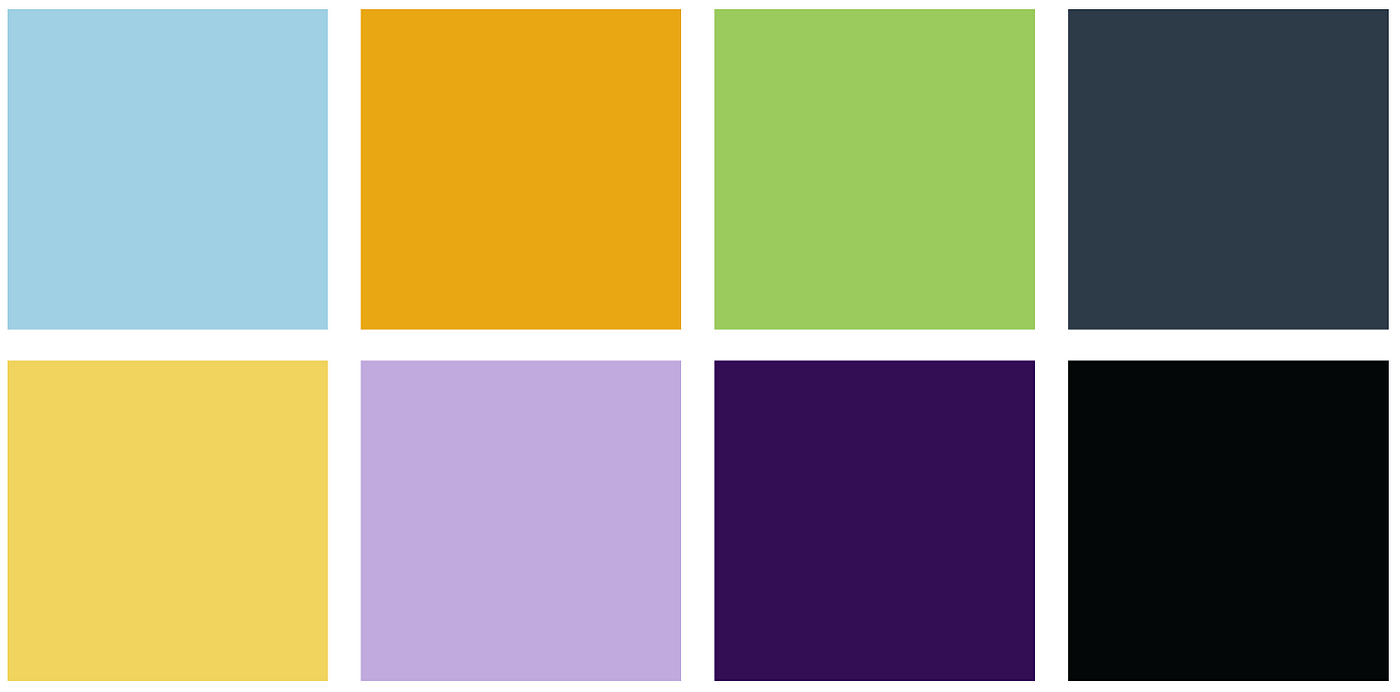
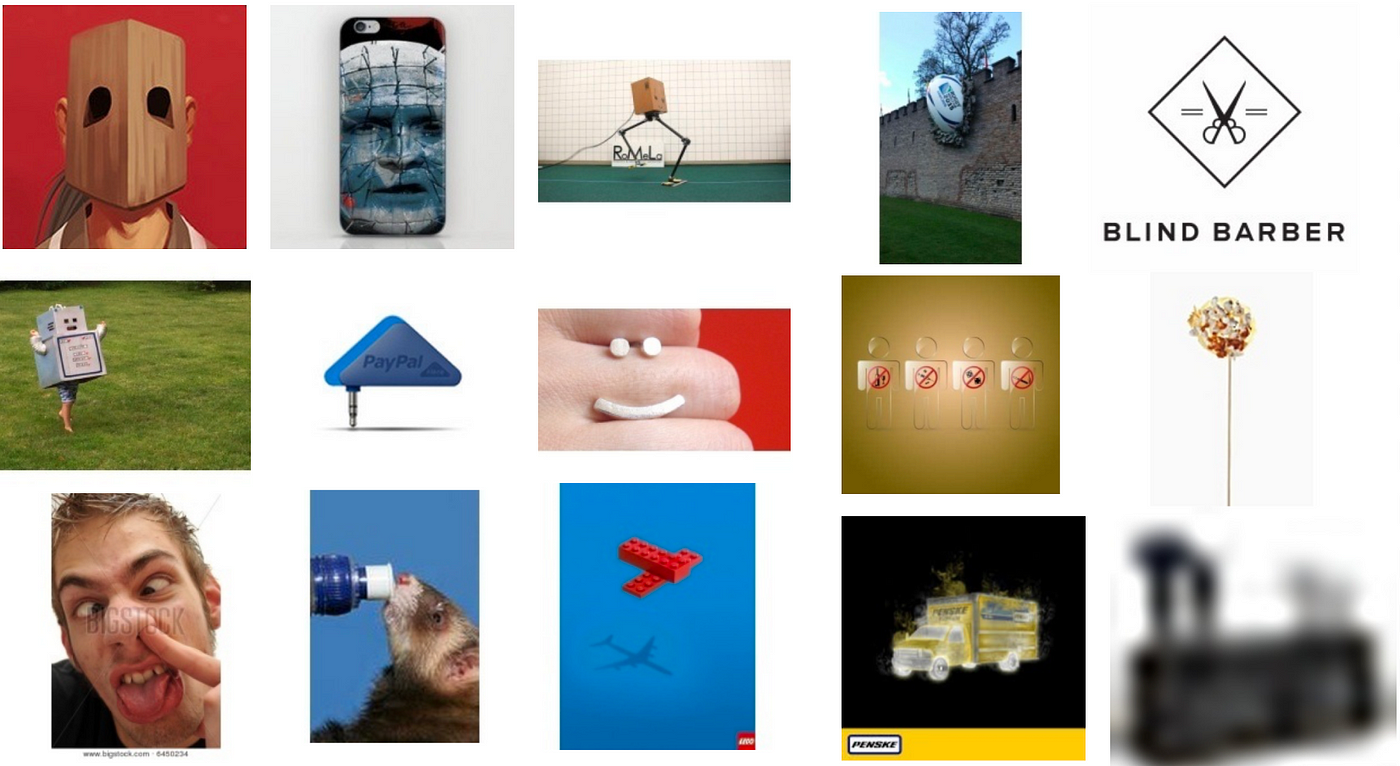
VL Datasets: Redefining Image Datasets
To address these challenges, we introduce VL Datasets — a collection of clean computer vision datasets.
VL Datasets are designed to minimize, if not eliminate issues such as duplicates, mislabels, outliers, data leakage, and more, transforming the way you engage with your datasets and ultimately, improving your model’s performance ².
Among the VL Datasets we’re proud to announce today is a cleaned-up version of LAION-1B, a dataset that is widely used for training generative AI models such as Stable Diffusion and Midjourney.
The following table summarized the issues found by analyzing various commonly used datasets.
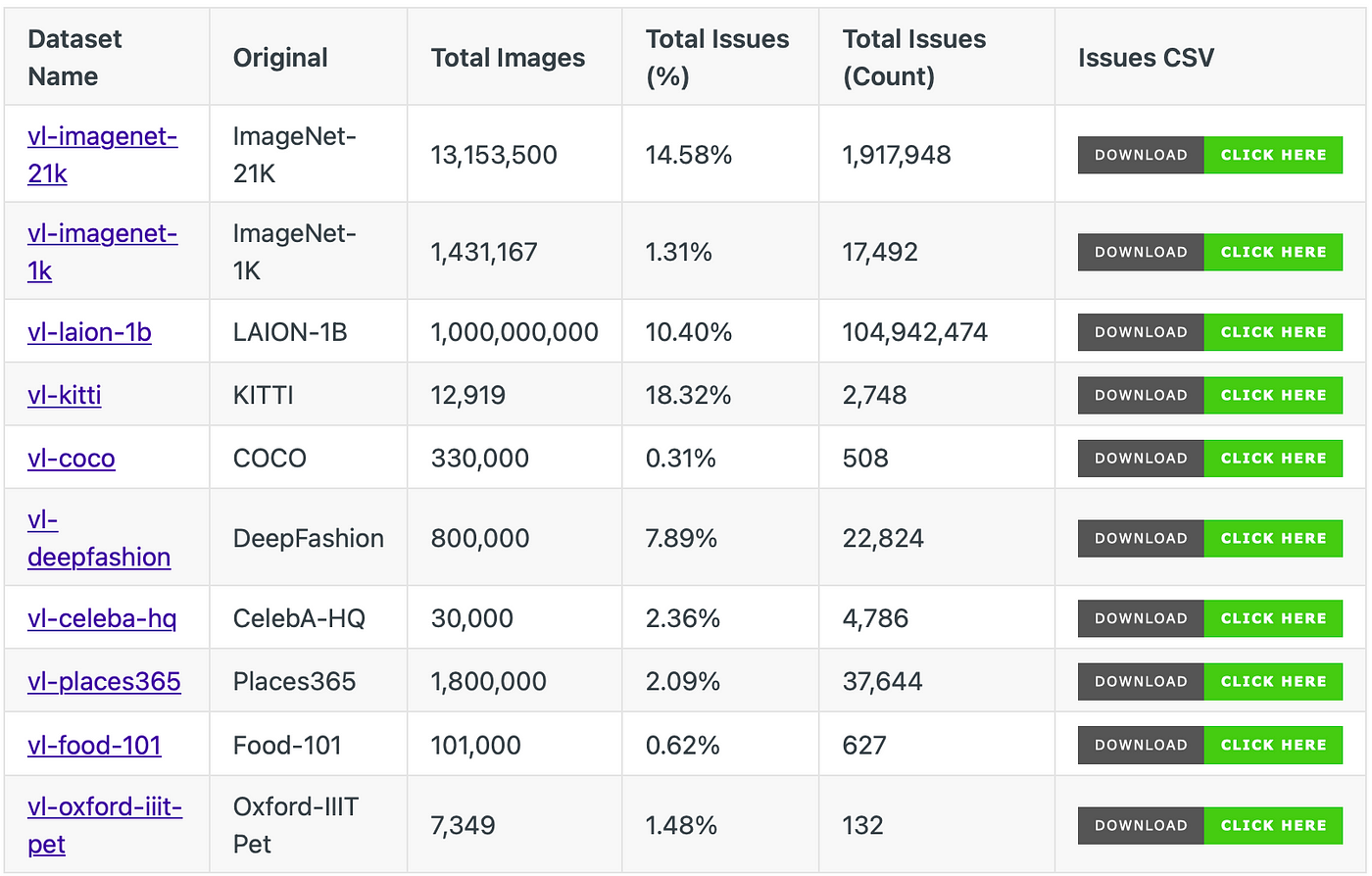
You can view a detailed breakdown of issues for each dataset on our documentation page and download the CSV files listing all problematic images for free.
How were VL Datasets Created?
VL Datasets were created by the Visual Layer flagship tool — VL Profiler. This advanced tool, based on usage from 220K users worldwide, utilizes a graph-based engine to identify and cluster data anomalies and quality issues, eliminating the need for tedious individual handling ³.
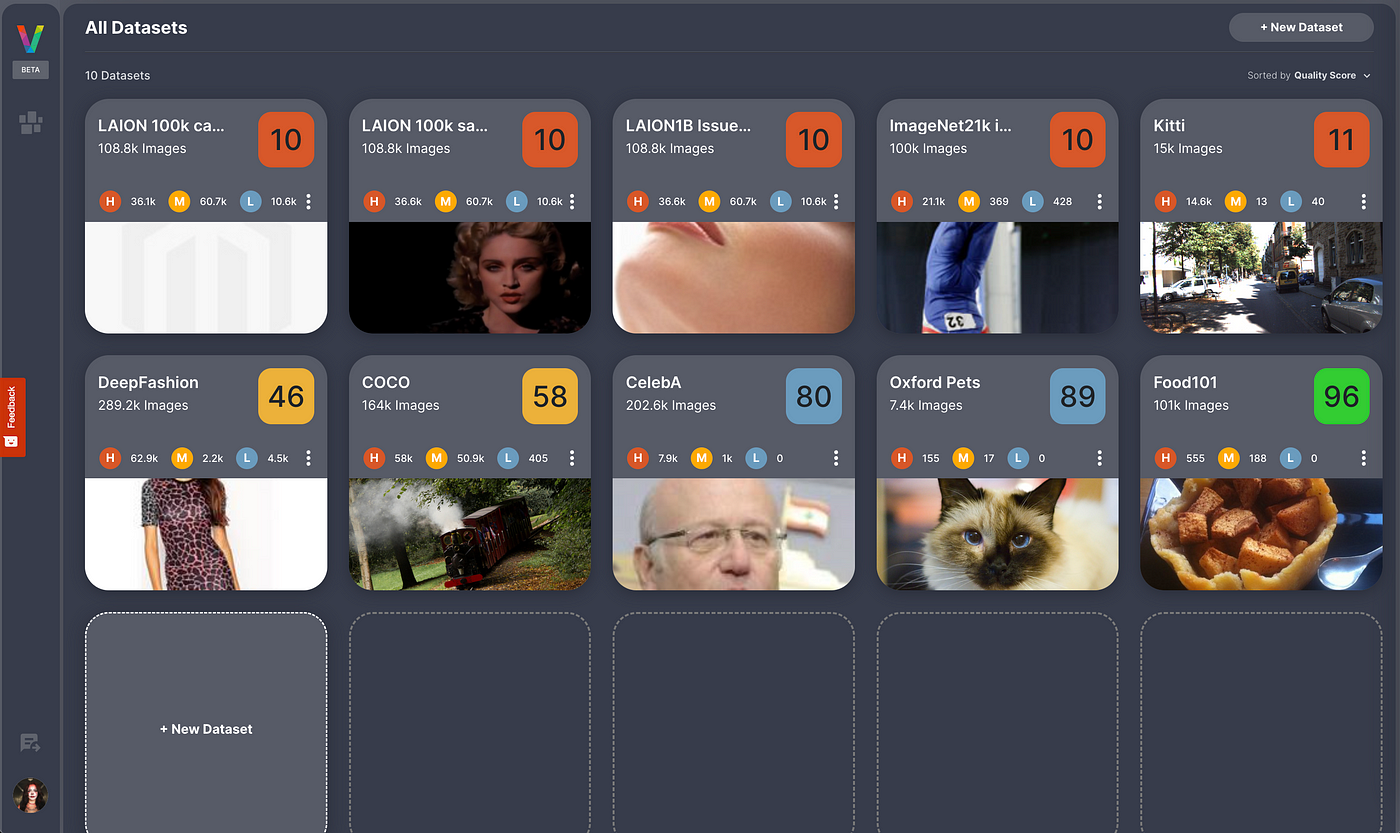
If you’d like to try VL Profiler on your own dataset and uncover potential issues, sign-up for free or visit our documentation to learn more.
Getting Started with VL Datasets
You can access VL Datasets using the open-source visuallayer
Python SDK OR using the VL Profiler, a user interface that runs in the browser.
To install the SDK, run:
pip install visuallayer
To load a dataset you’ll only need 2 lines of code:
import visuallayer as vl
dataset = vl.datasets.zoo.load('vl-imagenet-1k')
Once a dataset is loaded you can view the metadata with:
dataset.info
Metadata:
--> Name - vl-imagenet-1k
--> Description - A modified version of the original ImageNet-1k dataset removing dataset issues.
--> Homepage URL - https://www.image-net.org/
--> Number of Images - 1431167
--> Number of Images with Issues - 15997
Get a report showing a breakdown of the issues:
dataset.report
| reason | count | pct |
|------------|-------|-------|
| Duplicate | 7522 | 0.565 |
| dark | 3174 | 0.238 |
| blur | 2478 | 0.186 |
| Mislabeled | 1480 | 0.111 |
| Outlier | 1199 | 0.090 |
| leakage | 869 | 0.065 |
| bright | 770 | 0.058 |
| Total | 17492 | 1.313 |
You have 2 options to explore the issues in the dataset.
Option 1 — Exploring using the VL Profiler which offers extensive visualizations of your dataset and issues.
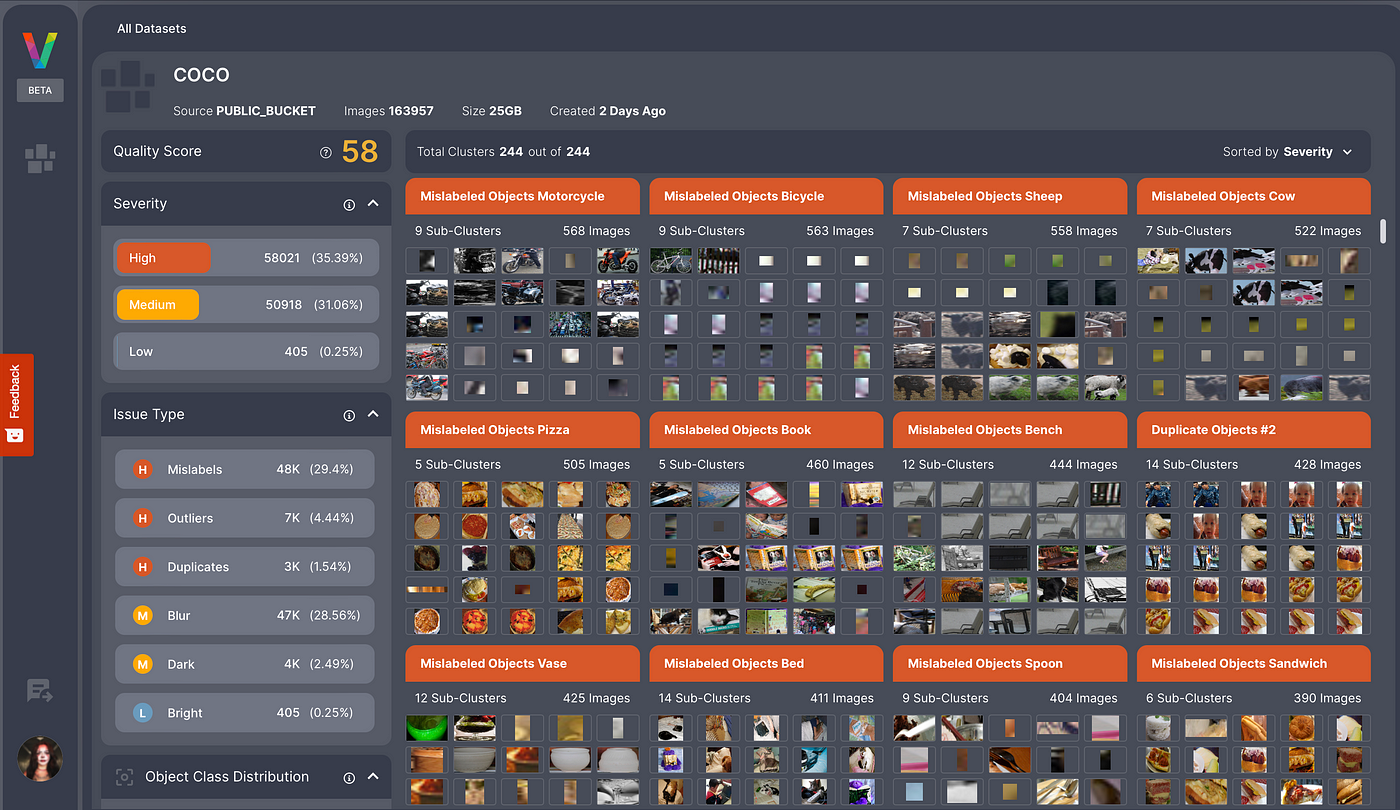
Option 2 — Exploring in Jupyter notebook using the SDK.
If you’d like to explore the issues in Jupyter notebook run:
dataset.explore()
This outputs an interactive table that lets you view, search, and sort the issues as shown below.
You can also export the dataset for training in PyTorch:
# Export to PyTorch
train_dataset = dataset.export(output_format='pytorch', split='train')
# Pytorch training loop
...
View a complete example from loading a dataset to training in PyTorch and fast.ai.
We created VL Datasets to be free and open-source as a way of giving back to the community. VL Datasets can be used as a starting point to train your machine learning model on clean datasets.
Conclusion
In the rapidly evolving field of AI, we believe that resources like VL Datasets are critical for driving advancements in Generative AI and other applications. By making the management of large-scale image datasets more efficient and effective, we hope to pave the way for more robust and reliable AI models.
We invite you to join us in our journey to enhance the quality of large image datasets and unlock the full potential of your data. To get started visit our GitHub repository or join our Slack channel for any enquiries.
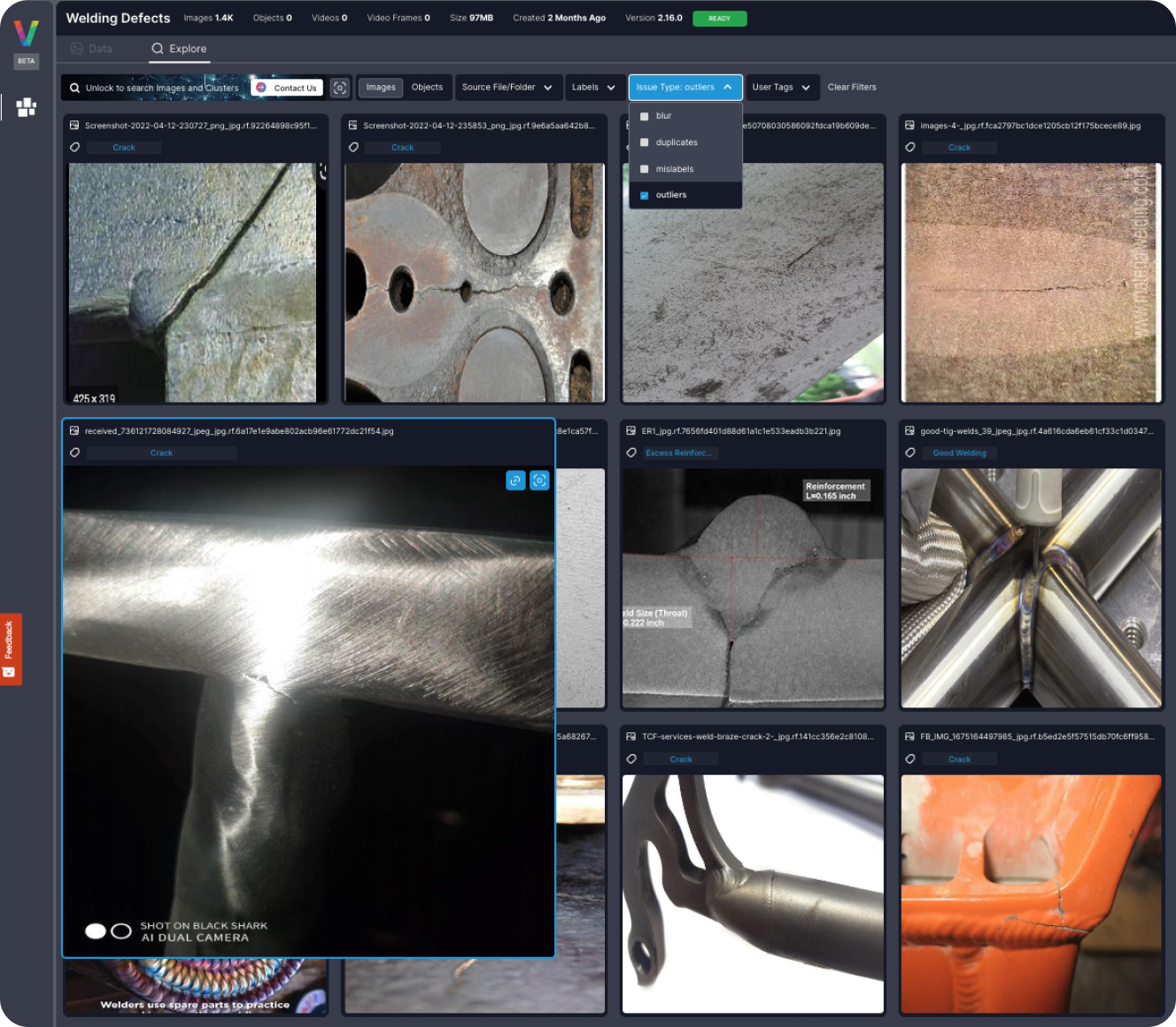
Introduction to Image Captioning
h2
h3
Image Captioning is the process of using a deep learning model to describe the content of an image. Most captioning architectures use an encoder-decoder framework, where a convolutional neural network (CNN) encodes the visual features of an image, and a recurrent neural network (RNN) decodes the features into a descriptive text sequence.
VQA
Visual Question Answering (VQA) is the process of asking a question about the contents of an image, and outputting an answer. VQA uses similar architectures to image captioning, except that a text input is also encoded into the same vector space as the image input.
code
Image captioning and VQA are used in a wide array of applications:
- point
- point
- point
Why Captioning With fastdup?
Image captioning can be a computationally-expensive task, requiring many processor hours to conduct. Recent experiments have shown that the free fastdup tool can be used to reduce dataset size without losing training accuracy. By generating captions and VQAs with fastdup, you can save expensive compute hours by filtering out duplicate data and unnecessary inputs.
quote
Getting Started With Captioning in fastdup
To start generating captions with fastdup, you’ll first need to install and import fastdup in your computing environment.
Processor Selection and Batching
The captioning method in fastdup enables you to select either a GPU or CPU for computation, and decide your preferred batch size. By default, CPU computation is selected, and batch sizes are set to 8. For GPUs with high-RAM (40GB), a batch size of 256 will enable captioning in under 0.05 seconds per image.
To select a model, processing device, and batch size, the following syntax is used. If no parameters are entered, the fd.caption() method will default to ViT-GPT2, CPU processing, and a batch size of 8.
“The captioning method in fastdup enables you to select either a GPU or CPU for computation, and decide your preferred batch size. By default, CPU computation is selected, and batch sizes are set to 8. For GPUs with high-RAM (40GB), a batch size of 256 will enable captioning in under 0.05 seconds per image.”
Dean Scontras, AVP, Public Sector, Wiz
FedRAMP is a government-wide program that provides a standardized approach to security in the cloud, helping government agencies accelerate cloud adoption with a common security framework. Achieving a FedRAMP Moderate authorization means Wiz has gone under rigorous internal and external security assessment to show it meets the security standards of the Federal Government and complies with required controls from the National Institute of Standards and Technology (NIST) Special Publication 800-53.
Image captioning and VQA are used in a wide array of applications:
- ⚡ Quickstart: Learn how to install fastdup, load a dataset, and analyze it for potential issues such as duplicates/near-duplicates, broken images, outliers, dark/bright/blurry images, and view visually similar image clusters. If you’re new, start here!
- 🧹 Clean Image Folder: Learn how to analyze and clean a folder of images from potential issues and export a list of problematic files for further action. If you have an unorganized folder of images, this is a good place to start.
- 🖼 Analyze Image Classification Dataset: Learn how to load a labeled image classification dataset and analyze for potential issues. If you have labeled ImageNet-style folder structure, have a go!
- 🎁 Analyze Object Detection Dataset: Learn how to load bounding box annotations for object detection and analyze for potential issues. If you have a COCO-style labeled object detection dataset, give this example a try.